Employee-centered Industry 5.0 - Case analysis of Haier
Abstract
How green digital intelligence and ecology promote enterprises to transform into industry 5.0 has attracted the attention of the industry and academia. Still, its internal driving mechanism at the micro level has not been clarified. Based on the perspective of enterprise dynamic capability and resource allocation, this paper studies the “path-process-capability” logical scheme of Industry 5.0 restructuring value chain. Firstly, the multi-case configuration analysis is carried out through focused stepwise quantitative case analysis to explore the resource collaboration path of Industry 5.0 transformation. Then, the process of digital intelligence and ecological reconstruction of the value chain is studied longitudinally for a single case, and the stage characteristics of green digital intelligence and ecology driving the Industrial 5.0 transformation of manufacturing enterprises are studied by combining the time series interval method and the critical event method. The process mechanism of digital intelligence and Industry 5.0 key capabilities are found. Aimed at the path problem of green digital intelligence and ecological reconstruction of the manufacturing value chain, based on multi-case statistical analysis and single-case in-depth analysis, the reconstruction mechanism and scheme of manufacturing ecological value chain were obtained. The research findings are as follows: firstly, four configuration forms of digital intelligence and ecological resource allocation are identified, and the rational allocation of resources is realized through the collaborative effect of resources. Secondly, the ecological process of green digital intelligence of manufacturing enterprises is based on the process of “standardization - online - integration - intelligence - ecological - Industry 5.0”, and the key technologies of each stage are identified. Third, in the transformation process of Industry 5.0, three key capabilities of enterprise digital production, digital drive, and ecological economy are constructed and data-led to promote the transformation and development of enterprise intelligent services and leverage the new business form of digital ecology.
Keywords
INTRODUCTION
Europe has proposed Industry 5.0 based on Industry 4.0, and according to the European Commission, Industry 5.0 implies a paradigm shift compared to Industry 4.0. Industry 5.0 originates from Industry 4.0, but it is not a simple continuation but rather focuses more on social and ecological values: it requires industrial production to respect and protect the earth’s ecology, place interests of workers at the center of the production process, and enable industry to achieve social goals beyond employment and growth, becoming the cornerstone of social stability and prosperity. Industry 5.0 has three core elements: human-centered, sustainability, and resilience[1]. This fundamentally differs from the system-based manufacturing that has pursued efficiency, quality, and cost reduction since Industry 1.0. Many scholars in European society consider Industry 5.0 to be the key direction of change for the manufacturing industry in the next decade. Based on the perspective of Industry 5.0, German companies have adjusted their smart manufacturing goals to a “smart service world”.
From the enterprise perspective, technology empowerment accelerates the value chain reconstruction of the traditional manufacturing industry, leading to new industry competition; from the social perspective, it optimizes the production process and improves efficiency and productivity while ignoring the “human” as the most important subject. How to shift the industrial focus from technology to human physical and mental health, natural sustainable development, and industrial resilience is a research question, and human-machine intelligent collaboration is the key to the future of human-centered intelligent manufacturing. However, it is not easy for the manufacturing value chain to transform into Industry 5.0, whose transformation goal is to upgrade the manufacturing value chain. Traditional manufacturing enterprises need to find a green digital transformation method that can enhance the core business competitiveness and create new business models. Strengthening the matching of green digital ecological technology with different development paths of enterprises is an urgent problem to be solved in the process of Industry 5.0 transformation. In the practice of Industry 5.0 transformation in the manufacturing industry, it is easy to fall into the following path dilemmas: (1) The green digital ecological reconstruction path of the value chain is equal to the informatization path. Many digital technologies involve a wider ecosystem and demand rather than just focusing on the internal information system of the enterprise. The value chain and the environment are compatible, which is the basis for the successful transformation of the enterprise; (2) The Industry 5.0 transformation path is limited to information technology (IT) innovation. GARTNER industry report database shows that more than 75% of enterprises simply start from the technology priority perspective and regard digital transformation as a means of investing in new technologies or IT construction. Most managers focus on the technical innovation problem, which is only a part of the enterprise transformation. The key problem is organizational management innovation. To break this misunderstanding, it is necessary to clarify that Industry 5.0 reconstruction includes not only technology transformation but also business and organizational transformation to fully integrate digital technology and supply chain and move from single innovation to diversified ecological innovation.
This paper takes the top-level design of Industry 5.0 transformation as the breakthrough point through multiple case studies of the general resource allocation path of manufacturing industry transformation, the single case study of transformation example, to find the feasible process of Industry 5.0 transformation reconstruction from the perspective of dynamic capability. The generation path of the value chain is derived from the production mode of the manufacturing industry, and then the analysis framework is constructed according to the dynamic capability theory and the value chain generation theory. The examples are used to analyze the key capabilities of enterprise transformation proposed by the dynamic capability, and the transformation path of Industry 5.0 is obtained. This article hopes to provide theoretical contributions to the transformation of Industry 5.0 and guidance for manufacturing enterprises to carry out green digital intelligence ecological construction.
REVIEW
Industry 5.0
Industry 5.0 focuses more on the importance of people. It is necessary to enhance individual skills and capabilities in collaboration or cooperation with digital technologies[2,3]. Meanwhile, Industry 5.0 (I5.0), the latest industrial revolution, addresses several limitations of its predecessor, I4.0. The latter was meant to improve organizational efficiencies and productivity through enhanced integration, automation, real-time data, and information exchange[4]. Some supporting technologies for Industry 5.0 include edge computing, digital twin collaborative robots, the Internet of Everything, blockchain, and 6G and beyond networks. Industry 5.0 centers on sustainable development; green operational practices play a crucial role in enhancing green manufacturing performance[5]. Green manufacturing refers to cutting-edge production techniques that use waste reduction, waste recycling, improved resource use, and other related strategies to lessen their negative environmental effects[6].
Industry 5.0 builds upon the current trend of Industry 4.0. The previous industrial revolutions brought about the use of steam, electricity, computers, and automation in manufacturing, and Industry 4.0 introduced digital technologies and the Internet of Things (IoT) to the production process. Industry 5.0 is anticipated to combine advanced technologies such as robotics, artificial intelligence (AI), and the IoT with human-centric design principles to create more efficient, flexible, and sustainable production systems[7]. It is an integration of resilient, sustainable, and human-centric technologies, organizational concepts, and management principles for designing and managing cost-efficient, responsive, resilient, sustainable, and human-centric value-adding systems at the levels of ecosystems, supply chains, and manufacturing and logistics facilities, data-driven and dynamically and structurally adaptable to changes in the demand and supply environment to secure the provision of society with products and services in a sustainable and human-centric way through the rapid rearrangement and reallocation of its components and capabilities[8]. One of its main drivers is the need for greater collaboration between humans and machines. As machines become more advanced and can perform more tasks, they will need to be designed to work seamlessly with human operators. In this way, machines can assist workers in tasks that require precision, strength, or speed, while humans can provide creativity, decision-making, and problem-solving skills[9]. Industry 5.0 also has the potential to greatly reduce waste and environmental impact in the manufacturing process. Smart factories can use data analytics to optimize production processes and reduce material waste while also implementing more sustainable energy sources and reducing greenhouse gas emissions[7].
Although machines and robots have successfully taken on heavy and repetitive tasks[10], humans remain indispensable in intelligent manufacturing. Their cognitive abilities and tacit knowledge are irreplaceable when handling flexible, unexpected, and uncertain manufacturing tasks. At the core of Industry 5.0 lies the human-cyber-physical system (HCPS), a composite intelligent system where interactions among humans, cyberspace, and physical assets are orchestrated across diverse manufacturing levels and phases[11]. The physical and cognitive capabilities of humans are enhanced by adopting wearable devices, human-machine interfaces, and advanced industrial big data analytics in industrial HCPS. The research on HCPS for Industry 5.0 is yet in its infancy.
The goal of Industry 5.0 is already clear, namely the smart manufacturing phase. Industry 5.0 hopes to make production processes digital, networked, and intelligent by applying emerging technologies such as IT, AI, and the IoT. It builds an intelligent, people-oriented, sustainable development of the industrial system, improves production efficiency and product quality, and promotes industrial development into a new stage. It emphasizes human-machine collaboration, combines human intelligence with machine accuracy, and achieves a higher level of automated production through technologies such as AI and machine learning while retaining human advantages in creative thinking, judgment, and decision-making ability. Current research on Industry 5.0 focuses on concepts and intelligent technologies but ignores the specific path for enterprises to carry out Industry 5.0 transformation. How to gradually cultivate key capabilities through enterprise-stage decision-making and resource allocation is the content we need to study next.
Green, digital and intelligent ecological transformation of enterprises
With the increasing development of digital technology, how to change the management of enterprises to meet the needs of the digital age has become the focus of current research when it affects the products, services, business processes, and collaboration methods of enterprises and changes the market potential. The realization of enterprise digital transformation needs the joint guidance of strategy and implementation framework and the organizational capacity building to ensure the strategy implementation. According to Matt et al.[12] and Hess et al.[13], there are four dimensions of digital transformation: technology use, value creation changes, structural change, and finance. In terms of organizational capabilities, dynamic capabilities become those focused on the enterprise transformation process. Warner and Wager proposed the significance of digital sensing, digital seizing, and digital transformation capability building[14].
Because digital technologies, such as big data, AI, and blockchain, can process, store, transmit, analyze, and connect large-scale data at extremely low cost, digitalization can change human labor, unconventional work, and cognitive processes[15]. At the same time, as performance improves, digitalization also brings conflicts and tensions. How to carry out digital transformation to help organizations alleviate conflicts and improve efficiency has become an important issue. Gastaldi et al. proposed in their study that three complementary paths are internal asset digitization, digital process integration, and disruptive decision-making through analysis, which can help improve the exploration-exploitation paradox[16]. In addition, the scope of enterprise digital transformation involves the strategic renewal of organizational business models, collaboration methods, and culture[14]. In the context of a wide range and deep transformation, enterprises need to balance existing capabilities and build new capabilities[17] to realize digital transformation. Enterprises need to build digital dynamic capabilities internally[13]. External needs to emphasize the integration of digital resources and technology integration capabilities, the construction of network capabilities, to avoid the problem of value co-destruction, form a new value co-creation behavior of customer value co-creation, supplier value co-creation, and build value co-creation network[18].
Under the influence of digital technology, the platform ecosystem emerges as a new form of inter-organizational relationship, which has an important impact on the mode and characteristics of enterprise innovation and entrepreneurship. The logic of value creation changes from the independent creation of a single enterprise to the value co-creation between enterprises in the ecosystem, and digital platforms and ecosystems have become a hot research field. This part of the research focuses on the impact of digital technology and platform ecosystem on innovation and entrepreneurship and the transformation of value creation logic. The basic characteristics of programmability and data homogeneity of digital technology have led to the emergence of innovation methods, such as platform, distributed, combinatorial, fusion, and generative innovation[19], which have changed the openness, support, and creativity of innovation and entrepreneurship[20], making innovation and entrepreneurship theory boundary restrictions and predefined constraints less[21]. Meanwhile, with the separation of information and material, service innovation is accelerated, and progressive innovations will be invisible and digital. In this context, Nambisan[21] put forward the concept of digital innovation, which refers to the creation of market products, business processes, or business models and the consequent changes generated using digital technology in the innovation process.
The emergence of the platform ecosystem supported by digital technology has made an important change in the way of enterprise value creation and delivery. In terms of resource access, digital platforms and ecosystems facilitate the availability and portability of open resources for enterprises of all sizes, transforming the resource ownership mindset to a resource orchestration mindset[22]. In value creation and delivery, digital technologies can transform an increasing number of physical products and services into digital forms through greater connectivity between digital platforms[23]. The hierarchical modular architectural features of products and platforms also support more flexible ways of value creation and delivery, enabling new business models and entrepreneurial initiatives. Therefore, to fully play the role of participants in the platform ecosystem in new value creation and delivery methods, enterprises should use different IT support capabilities for various types of participants. The targeted use of digital technology is more important than the blind pursuit of the full deployment of digital technology.
Digital technology is deeply embedded into the products and services of enterprises, which promotes the change of value-dominant logic from product-dominant logic to service-dominant logic, and digital servitization has become a hot research field. This part of the research mainly discusses the change in products and services under the influence of digital technology, how this change affects enterprises, competition, inter-enterprise relations, and the phenomenon of digital hermitization of enterprises based on service dominance logic and its value mining. Different from the changes brought by IT in the past, intelligent and connected products formed by combining digital technology and products support new product functions and capabilities, which can realize condition monitoring, function control, performance optimization, and autonomous operation functions. Customer value positioning has also shifted from acquiring independent products to acquiring digital solutions, and the value-dominated logic has gradually shifted from product-dominated logic to service-dominated logic centered on tangible and intangible market services. An expanding array of enterprises begin to transform to service at the operational and strategic level through path and dynamic resource allocation to increase their competitive advantages[24]. The transformation of the nature of products and services and the dominant logic of value has internally affected enterprise operation, organizational structure, and business model and externally changed the supply chain relationship, industry structure, and nature of competition[25].
As the value of digital servitization has been widely affirmed, more researchers have begun to discuss the requirements and stages of enterprise digital servitization transformation. From the perspective of relationship theory, Kamalaldin et al. proposed four key relationship elements that are complementary digital capabilities, relational-specific digital assets, digitized knowledge-sharing procedures, and cooperative governance to profit from digital servitization[26]. Kohtamäki et al. propose that in addition to digital technologies, the transformation to advanced services requires new organizational processes and resources, which need to be captured through new business models[27]. Paola and Marco proposed three business models of digital servitization in manufacturing from the perspective of transformation scope, namely product-, process-, and result-oriented digital servitization[28]. Chen et al. proposed that digital servitization needs to go through three stages from the perspective of transformation results, from the stage of providing standard products and services, to the stage of providing customized solutions, and to the stage of providing intelligent solutions with ecosystems[29]. The enterprise value proposition, delivery, and capture mechanisms change with the evolution of the stage.
A business model is defined as the architecture of an enterprise’s mechanism for creating, delivering, and capturing value[30]. The empowerment of digital technology, the transformation of value creation logic and value dominance logic, and the new inter-subject relationship and competition environment promote the transformation of the enterprise business model to the digital service model. The reason the digital transformation of enterprises is closely related to the business model is first based on the importance of the business model itself. Internally, business models create the link between strategy and implementation[31]. Externally, the business model, as a system of interrelated activities, determines the relationship between enterprises and customers, partners and suppliers; Secondly, a close relationship exists between business model and technology, and business model plays an important role in the value mining and market results transformation of digital technology[32]. Business model choices affect how technology is developed, how technology is monetized, and the profitability of the company[33]; Finally, the digital economy poses a major challenge to traditional business models[34], and enterprises must change and develop new business models to adapt to the new environment changes. Business model innovation is defined as the implementation of novel, significant changes to key components of a company’s business model or the architecture that connects these components, in response to strategic discontinuity and intense global competition[35]. Among them, innovative enterprise digital servitization business models enable new value creation and capture through the monitoring, control, optimization, and autonomy of intelligent solutions or intelligent product service systems[36].
The essence of the problem of business model innovation and change is growth in a social context[37]. Continuous and rapid product iteration, response to environmental changes, and opportunistic behavior to expand into new markets are important factors for enterprises to initiate business model innovation. The development of digital technology is an important factor in promoting the reform of business models. The expansion of the scope of business networks and the deepening of the participation of relevant subjects brought by digital technologies, such as blockchain and 3D printing, have sharply increased competition and accelerated the speed of business model innovation[38]. Digital technologies, such as big data, the IoT, and AI, provide massive information[27] and analytical and predictive technologies[39] for the transformation of business models into digital services. With the development trend of intelligent interconnection and service, more consumption scenarios and demands have emerged, and consumers increasingly rely on personalized solutions based on digital technology to meet their own needs.
RESEARCH DESIGN
Research methods and cases selection
The core content of this paper is to study the internal mechanism of green digital intelligence and ecology driving the Industrial 5.0 transformation and upgrading of manufacturing enterprises, focusing on the path of green digital intelligence and ecological reconstruction of the manufacturing value chain, aiming to explore how manufacturing enterprises use green digital intelligence and ecological reconstruction to realize the Industrial 5.0 transformation and upgrading from the perspective of dynamic capabilities and resource orchestration. The configuration analysis of multiple cases is carried out through focused stepwise quantitative case analysis (fsQCA) to explore the collaborative path of Industry 5.0 transformation resources. The fsQCA method is used because (1) Green digital intelligence promoting Industry 5.0 is a complex process, and the factors affecting the ecological level of digital intelligence are interdependent. This paper hopes to horizontally study multiple antecedent conditions (profitability, R&D expense ratio, growth ability, company size, human capital, employee) through case studies on how satisfaction, environmental, social, and governance (ESG), and digital strategy affect the ecological level of enterprise digital intelligence, the antecedent conditions are concurrent, and it is suitable to use fsQCA for research; (2) fsQCA can solve the same path problem well. By comparing the similarities and differences between different configurations that lead to the result, it can analyze various paths. Using fsQCA, diverse paths leading to the ecological level of enterprise data intelligence can be compared and analyzed; (3) fsQCA has a lower requirement on sample size and is especially suitable for analyzing small and medium-sized samples. Therefore, it is selected for the multi-case horizontal study in this paper.
The transformation of enterprise Industry 5.0 is a process with complex and dynamic factors, which involves the changing and interlaced influence of the industrial competition environment and technological change in different time stages. Through the vertical in-depth analysis of the case situation, the complex problems can be better dissected, the hidden theoretical logic behind it can be excavated and the theory can be condensed. It is feasible and compatible to find the transformation and reconstruction path and key capabilities of Industry 5.0 from the perspective of dynamic capabilities from the green digital intelligence and ecological practice case of Haier Lighthouse Factory. The reasons are as follows: (1) After years of development, Haier Group has become an industry-leading green, digital, intelligent, and ecological enterprise from a small collective household appliance factory, which is representative in related fields and conforms to the theoretical sampling principle; (2) Haier has a high level of digital intelligence ecology, which is a model of Industry 5.0 and holds research value. Haier Lighthouse Factory represents the most advanced intelligent manufacturing factory in the world. In addition, its “integration of people and orders” management mode has been highly recognized by the global management academia and the business community, and it is also believed that it may become the next social model, in line with the extreme principle; (3) Our team has been paying attention to Haier for a long time and has conducted in-depth investigations many times, mastering abundant data and materials, which is in line with the principle of data richness.
Data collection
This paper collects Haier Lighthouse Factory enterprise field research data, executive interview data, listed company annual reports, and industry analysis reports and obtains various data by consulting newspapers, magazines, news reports, research reports, website information, corporate annual reports, academic papers, wind database, and other methods; from the perspective of statements and external authoritative evaluations of internal managers, text mining and verification are carried out to improve the confidence and reliability of case studies.
The case selection considers the principles of representativeness and uniqueness. In terms of time, Haier Lighthouse Factory’s digitalization and greening transformation is ahead of the industry, and its experience can reflect the mechanism and details of green digital ecological transformation more completely, providing rich data on the transformation process. The main procedures of data analysis are as follows: (1) encode and analyze the original data and extract the evidence of the enterprise upgrading stage and collaborative value creation; (2) refine the relevant evidence, summarize the corresponding relationship between system collaboration characteristics and ecosystem stage; (3) combine the relevant theoretical research, conduct axial coding, propose propositions, and form research conclusions; (4) present case evidence from three perspectives: case, text, and speech, to ensure the accuracy of the conclusions.
The case study is feasible. The research team has long-term project cooperation with Haier Lighthouse Factory and provides IT planning solutions for its digital ecological and green development in the consulting project and has a sufficient grasp of its basic situation and key events. The research team has conducted research interviews with the company and its ecosystem enterprises, covering the grassroots, middle, and senior levels, laying a solid data foundation for an in-depth discussion of their interaction process, identifying key issues in the transformation, and refining theoretical innovation.
STUDY 1: MUTI-CASE FSQCA ANALYSIS AND DISCOVERY
Qualitative comparative analysis (QCA) is a case-oriented research method that is suitable for small and medium-sized samples. This strategy adopts a holistic perspective, focusing on the comprehensive explanatory power of different combinations of condition variables for the outcome variable, and can identify different condition configurations that lead to the same outcome. Therefore, QCA is very useful for identifying the complex antecedents of digital transformation.
Data description
Based on the dynamic capabilities and resource orchestration theory, this paper selects enterprise profitability, R&D expense ratio, growth ability, company size, human capital, employee satisfaction, enterprise ESG, and management layer digital strategy as antecedents, and the three key indicators of industrial 5.0 transformation (human-centered, sustainability, and industrial resilience) as consequences, to study the comprehensive index of digital ecological level. The data used in this paper comes from the Wind database and the public annual reports of various enterprises, and the time is 2022. The paper selects large, medium, and small manufacturing enterprises from the Growth Enterprise Market and the New Third Board, involving different levels of the manufacturing industry, and excludes cases with incomplete data, resulting in a medium-sized sample size of 39. Secondly, the selected enterprises are all of the types that use digital technology to transform their original production, management, sales, etc., highlighting the research purpose of digital technology empowering the manufacturing industry. The specific condition measurement methods are shown in Table 1.
Quantitative method of antecedent factors
Antecedent factors | Quantitative method |
Profitability | Return on equity (average) (%) |
R&D expense ratio | R&D expenses/Total revenue (%) |
Growth ability | Total revenue growth rate (%) |
Company size | Total revenue (ten thousand yuan)/Total number of employees |
Human capital | The ratio of undergraduate, master and doctoral to employees |
Employee satisfaction | Questionnaire rating |
ESG | Wind ESG rating |
Digital strategy | Digitize the text of the annual report of the enterprise |
Data calibration
Following Fiss’s approach[40], we calibrate the fuzzy sets of enterprise profitability, R&D expense ratio, growth ability, company size, human capital, and enterprise digitalization level: we set the three calibration points of full membership, crossover point, and full non-membership as the upper quartile, the mean of the upper and lower quartiles, and the lower quartile of the case sample, respectively. We use the fsQCA4.0 software to calibrate the data, transforming it into set memberships ranging from 0 to 1. The calibration points are shown in Table 2.
Variable calibration results
Variable | Point | ||
Full membership | Crossover point | Full non-membership | |
Profitability | 24.46 | 11.93 | -6.9 |
R&D expense ratio | 11.89 | 5.04 | 0.25 |
Growth ability | 96.19 | 6.73 | -18.84 |
Company size | 567.54 | 133.5 | 39.3 |
Human capital | 69.96 | 33.15 | 8.82 |
Digitalization level | 5 | 3 | 1 |
Single-condition necessity analysis
Using the fsQCA4.0 software, we performed a consistency analysis to obtain the consistency scores of each single variable for the outcome variable [Table 3]. The consistency scores of all condition variables for the outcome variable of transformation and upgrading did not exceed 0.9, indicating that none of them was a necessary condition for transformation and upgrading. The explanatory power of single antecedent variables for digital transformation was not strong.
Results of necessity analysis
Antecedent factors | High level of digitization | Antecedent factors | Low level of digitization | ||
Consistency | Coverage | Consistency | Coverage | ||
Profitability | 0.749881 | 0.743505 | ~Profitability | 0.540257 | 0.636007 |
R&D expense ratio | 0.664126 | 0.6867 | ~R&D expense ratio | 0.591234 | 0.663636 |
Growth ability | 0.685088 | 0.807865 | ~Growth ability | 0.659362 | 0.65283 |
Company size | 0.592663 | 0.709236 | ~Company size | 0.699857 | 0.684529 |
Human capital | 0.653645 | 0.762646 | ~Human capital | 0.610291 | 0.60971 |
Conditional configuration path analysis
Following Fiss’s approach[40], we set the consistency threshold to 0.8, the case frequency threshold to 1, and manually adjusted the outcome data with RPI less than 0.7 to 1 in the truth table. The specific results are shown in Table 4, which presents four configuration paths for the digital transformation of manufacturing enterprises, composed of five antecedent factors under the dynamic capabilities and resource orchestration theory.
Results of the digitized horizontal configuration analysis
Antecedent factors | Configuration paths | |||
a | b | c | d | |
Profitability | ○ | ▲ | ● | Δ |
R&D expense ratio | ○ | ▲ | Δ | ● |
Growth ability | ● | Δ | ▲ | ▲ |
Company size | ○ | ● | ● | ● |
Human capital | ● | ● | ▲ | ▲ |
Raw coverage | 0.24202 | 0.29395 | 0.281086 | 0.249166 |
Unique coverage | 0.0123868 | 0.0471653 | 0.0281086 | 0.01191 |
Consistency | 0.969466 | 0.898108 | 0.89939 | 0.944043 |
Overall coverage | 0.393044 | |||
Overall consistency | 0.891892 |
Growth-oriented small enterprises’ industrial 5.0 transformation path (configuration a)
Growth-oriented small enterprises are in the initial stage, with the characteristics of small company size, low revenue ability, and inability to invest (a certain amount of) capital in R&D. Digital transformation has a long cycle and high cost, and the lack of funds and high cost are important reasons that restrict small- and medium-sized enterprises from carrying out digital transformation. These types of enterprises need to devote themselves to the steady development of the company (that is, creating a certain growth rate of total operating income) as the basic condition, stabilizing the company’s survival ability and seeking development. At the same time, they need to improve the level of human resources, attract talents with high-quality talent development strategies, grasp the opportunities of digital transformation, and promote digital transformation.
Stagnant medium and large enterprises’ industrial 5.0 transformation path (configuration b)
Stagnant medium and large enterprises have a certain company size, profitability, and talent resources, but they are in a bottleneck period in the development process, and it is difficult for the company to increase its revenue to a higher level. There are situations where resources are invested but the digital transformation effect is not good. These types of enterprises have sufficient resources (funds and human resources) and resource investment behavior, but there is a problem of unclear output effect. The key for these enterprises is to improve the company’s profitability, on the one hand, to allocate the company’s funds reasonably, to evaluate the investment projects reasonably, and to improve the net profit rate of assets; on the other hand, to manage the production scientifically in the production process, reduce costs, and increase efficiency. Revenue creation is a necessary condition for R&D behavior. While reducing costs and increasing efficiency, the revenue-creating resource ability can be used to increase R&D investment and increase the R&D intensity in capital investment, which is expected to break through the bottleneck. R&D behavior is a risky behavior with large investments and uncertainty. Some enterprises have risk resistance mechanisms in the R&D process, which leads to insufficient R&D investment and poor innovation benefits. The innovation behavior of enterprises also works to reduce costs and increase efficiency, improve enterprise profitability, and have a certain consistency. The digital transformation path of stagnant medium and large enterprises is to use their company size and human resource level of resource ability, enhance enterprise revenue ability, increase R&D investment, and complement each other.
R&D-deficient enterprises’ industrial 5.0 transformation path (configuration c)
The characteristics of R&D-deficient enterprises are medium-sized companies with average company size and profitability, and little or very little, or even no R&D investment. In the market, enterprises use innovative R&D to drive revenue creation. Some enterprises have their R&D departments or cooperative forms of research units, but others do not have R&D departments (institutions). There are various behavioral reasons for the lack of R&D behavior or low R&D investment. For some enterprises, this is due to objective reasons such as economic ability and manager resource allocation. For others, the reason is that they have not conducted technological innovation for a long period after the transformation of the external results. For these types of enterprises, in the case of a lack of R&D investment, the key is to devote themselves to developing the company’s growth ability and improving the level of human resources. Stabilizing and enhancing the enterprise’s growth ability can be achieved through market positioning and strategic planning, sound financial management, continuous improvement, learning, etc. At the same time, another key is the accumulation of human resources level. Enterprises have sufficient high-quality talents, which can lay the foundation for digital transformation and promote the improvement of the digital transformation level. In the digital age, under the development opportunities of intelligent manufacturing, enterprises improve the level of human resources, which is the synergy of improving enterprise growth ability and stabilizing profitability and company size. The path configuration for enterprises with R&D behavior deficiency is to focus on the synergy of growth ability and human resource level while stabilizing company profitability and size.
Low-profit enterprises’ industrial 5.0 transformation path (configuration d)
In the market, enterprises may face product value, price competition, poor sales, and other single or compound reasons, resulting in low-profit problems, resource revenue mismatch, and poor enterprise efficiency. The capital investment of digital transformation may overwhelm the enterprise, but the successful transformation will also change its predicament and obtain new development, producing huge benefits. The key lies in the enterprise resource allocation in the transformation. For these low-profit enterprises with survival ability, the key to digital transformation is not too much R&D investment, which will lead to the heavy burden and unreasonable resource allocation of the enterprise. The essence lies in the resource tilt of improving the enterprise’s growth ability and talent resource level, and the company size expansion and R&D investment should be appropriate. The main business income of the enterprise increases continuously, which can continuously improve profitability and obtain profits, provide funds for purchasing digital equipment, developing digital products, and upgrading the process digitally, thus promoting the further development of digital transformation, highlighting its very importance for the enterprise. The exertion of digital technology requires human capital with certain skills. Talents are the implementers and promoters of enterprise digital transformation. The digital transformation path of low-profit enterprises is to leverage digital transformation through the resource tilt of enterprise growth ability and talent resource level.
STUDY 2: SINGLE CASE ANALYSIS AND DISCOVERY
A multiple-case analysis reveals the general path of resource allocation for industrial 5.0 transformation, and Haier (calibrated, profitability: 0.77, R&D investment: 0.33, growth ability: 0.5, company size: 0.66, human capital: 0.5) is a very successful case of digital and ecological transformation, which fits path b in the current stage of resource allocation. Haier Group, as the leader in smart home appliances, is outstanding in profitability and R&D investment. The macro path is insufficient to guide the digital transformation process, and this part focuses on the process and mechanism of green digital and ecological transformation, which reveals the digital and ecological process in depth, to answer the core question of “How green digital and ecological transformation drives the transformation of manufacturing enterprises”.
The stages of Haier’s transformation
Haier, as one of the most successful representative enterprises of industrial 5.0 transformation in the manufacturing industry, will bring enlightenment to more traditional enterprises in the digital transformation battle by combing through the key points and difficulties of its transformation process. This enterprise now has ten global R&D centers, 108 manufacturing plants, and 66 marketing centers, and has become the world’s leading home appliance brand, ranking among the world’s top 500, winning the “world’s largest home appliance brand” for nine consecutive years, and the “world’s largest home appliance brand retail volume” for ten consecutive times, which are the results of its first implementation of digital transformation. Since its establishment in 1984, it has gone through five strategic stages: brand, diversification, internationalization, globalization brand, and network strategy, and now enters the ecological brand strategy and intelligent service strategy [Table 5].
Haier Lighthouse Factory development strategy
Time | Strategy | Target | Resource allocation |
1984-1992 | Brand strategy | TQM | Vertical integration |
1992-1998 | Diversification strategy | SBU | Horizontal integration |
1998-2005 | Internationalization strategy | Market chain | Horizontal integration |
2005-2012 | Globalization brand strategy | “Rendanheyi” | Horizontal integration |
2012-2019 | Network strategy | Industrial internet | Platform integration |
2019-2022 | Ecological brand strategy | Platform ecosystem | Platform integration |
2023 | Intelligent service strategy | Industry 5.0 | The six elements of “RenDanHeY” |
In the realm of digitization, Haier embarked on its journey into the network landscape as early as 1995. Subsequent milestones include the year 2007 when the company transitioned towards informatization, followed by the establishment of the interconnected factory in 2012 and the launch of the Kairos platform in 2017. Central to Haier's transformational endeavors is the intelligent home practice, where the entire spectrum of processes, spanning product design, intelligent manufacturing, sales, post-sales services, and logistics, undergoes a comprehensive digital transformation.
Standardization (1995-2006) and online (2007-2011)
Regarding the status of Lean and Six Sigma quality system construction, Haier has developed certain Lean IE and Six Sigma management capabilities. Examples include using the New Product Nine-Step Management Model [Figure 1] and industrial knowledge mapping (DFMEA, PFMEA, MCP).
In production, Haier Smart Home has implemented a modular system. Initially, in the company’s product research and design, a standardized approach is adopted, reducing repetitive work, and integrating and unifying the company’s module and platform libraries. In manufacturing, modules are assembled and modified according to customer needs, thus meeting customer demands for personalized product customization and achieving zero inventory.
In 2008, Haier smart home’s Haier global value-added system (HGVS) was officially put into operation, marking a pivotal milestone in the reorganization of critical business processes and the reform of essential information systems. The deployment of the enterprise resource planning (ERP) system, a substantial improvement from past domestic management systems, facilitated the comprehensive integration of enterprise resources, encompassing an extensive array of processes and aggregating intricate business projects.
Within the domain of product management, a systematic approach is adopted through the utilization of the Product Lifecycle Management (PLM) system. This encompasses the entire process from initial product planning, through product development, refinement, sales, and post-sales services, to updates and upgrades. Emphasis is placed on a comprehensive understanding of product-related performance, value, and maintenance aspects. This knowledge is then leveraged to enhance product design, ultimately optimizing product quality and technological sophistication.
In the realm of external customer interactions, leveraging Internet technologies is integral to establishing a robust service infrastructure. Services related to product purchase, maintenance, and after-sales support are seamlessly addressed through online solutions, including handling financial data of online transactions. Furthermore, in the supply chain, a unified display interface for suppliers to identify discrepancies, automate inventory management, and optimize the ratio data for future shipments.
Integration (2012-2016)
Starting in 2012, Haier formally initiated the implementation of its network development strategy, marking the commencement of its exploration into the path of digital transformation. During this phase, it transitioned from traditional production models to large-scale customization, establishing interconnected factories to achieve a platform-oriented enterprise.
Zhang Ruimin introduced the concept of interconnected factories, emphasizing the need to connect suppliers and equipment. The Smart Manufacturing Project Team led the execution of the project, repeatedly dismantling and rebuilding the Shenyang factory as a basis to explore and construct interconnected factories. Over the four years from 2014 to 2017, based on the exploratory experience of the Shenyang Refrigerator Interconnected Factory, Haier summarized this experience into the “Interconnected Factory Model”. Subsequently, it successively established seven interconnected factories, including the Zhengzhou Air Conditioner Factory, Foshan Drum Washing Machine Factory, Qingdao Water Heater Factory, FPA Motor Factory, Jiaozhou Air Conditioner Factory, and Central Air Conditioner Factory. The interconnected factories utilize a multitude of intelligent sensors to establish connections between devices, soften production lines, intelligently differentiate between product models, and construct a smart production model.
Integration, as manifested in actual production, hinges on the efficient and cost-effective collection of diverse and heterogeneous data and the application of the collected data. In Figure 2, it can be seen that taking a discrete manufacturing enterprise as an example, industrial big data finds wide applications in (a) Virtual simulation, including digital twins and process simulation, along with real-time work measurement combining radio-frequency identification (RFID) and visual technology; (b) Automated transport and flexible production lines, incorporating product automatic tracking with RFID technology-based automated guided vehicle (AGV) and rail guided vehicle (RGV); (c) Automated production, encompassing industrial robots, intelligent error prevention, and quality traceability; (d) Visual recognition, involving industrial camera recognition, barcode recognition, AI vision, etc.; (e). Smart security, including face recognition and fire hazard warnings; (f) Smart energy, covering energy monitoring and alert management; (g) Intelligent maintenance, featuring predictive maintenance and virtual collaborative maintenance combining virtual reality (VR) technology; (h) Intelligent warehousing, with smart inventory and in/out operations using RFID technology; (i) Big data applications and user experience.
Intelligentization (2017-2019)
Haier’s entire customized production system includes outreach customers, mainly module customization, module setting, user design, online visualization and self-interaction, exclusive customization, complete personalization, and design effect visualization. It also includes the inline supply chain, mainly including real-time tracking and visualization of order transaction status, real-time tracking and visualization of origin and production status, real-time tracking and visualization of distribution information and logistics status, and visualization of user evaluation landing in the workshop.
Haier has strategically optimized production processes for different manufacturing types within the customized production framework. Furthermore, leveraging data models for process analysis not only provides managers with additional critical information for informed decision-making but also establishes a comprehensive management system that incorporates multi-dimensional and multi-level data sources, including production status, equipment status, implementation process parameters, and production cycle times.
Utilizing big data analytics, Haier has achieved intelligent and personalized custom production [Figure 3].
Figure 3. Practice ring of industrial big data. AMVC: Agile Manufacturing Value and Control Management; KPI: Key Performance Indicator; IoT: Internet of Things.
Data collection. The initial step involves gathering industrial big data from operational smart factories, utilizing the Cyber-Physical System (CPS) and IoT technologies. This includes data from workshop equipment, warehouses, offices, etc., encompassing production cycle time (Takt), process parameters, equipment parameters, buffer, Work-In-Process (WIP), waiting time, material flow, information flow, and process flow.
First, agile Manufacturing Value and Control Management. The second step entails constructing agile manufacturing value and control management, creating data models, and generating effective information outputs; Second, Key Performance Indicator (KPI) Determination and Analysis. The third step involves employing predefined KPIs and establishing auxiliary analytical tools for agile KPIs, enhancing real-time and precise performance analysis; Third, correlation Analysis and Process Control. The fourth step utilizes data from various sources, preprocesses process and equipment parameters, conducts appropriate data preprocessing on different independent and dependent variables, automatically performs correlation analysis to identify key factors, and implements process control and automatic iterative monitoring; Fourth, decision Analysis and Improvement. The fifth step involves managers optimizing data models and KPIs, analyzing simulation results, and making improvement decisions based on experience. After confirming the improvement direction, agile value stream analysis and hypothesis testing are conducted through data simulation, comparing improvement results with corresponding KPIs to inform improvement decisions; Fifth, detailed Improvement Design and Implementation Feedback. The final step entails a detailed improvement plan design and feedback of the plan to be implemented in the smart factory. This step follows a closed-loop improvement process, aligning with traditional Define, Measure, Analyze, Improve, Control (DMAIC) analysis.
Ecologization (2019-2022)
In 2017, Haier officially launched the COSMOPlat platform, a manifestation of its success in intelligent manufacturing and an industrial Internet platform that aligns more closely with the digital era after experiencing smart manufacturing and ecological platforms. This platform development signifies the initiation of knowledge management and experience sharing. The intelligent factory underwent significant improvements and upgrades, culminating in establishing the world’s first “Intelligent + 5G” interconnected factory in 2019, seamlessly integrating AI with fifth-generation mobile communication technology to achieve unparalleled control over all business processes.
COSMOPlat, introduced by Haier Group, is a national-level “cross-industry and cross-disciplinary” industrial Internet platform crafted based on over three decades of manufacturing experience. Positioned as an industrial Internet platform that involves users throughout the entire process, it provides scenario-based digital transformation solutions for enterprises of varying sizes and industries globally. It propels the transformation of production methods, business models, and management paradigms, fostering the widespread adoption of new models and formats while constructing an industrial new ecosystem characterized by co-creation, sharing, and high-quality development in the realms of “government, industry, academia, research, application, and finance”.
Within the business sectors, COSMOPlat covers industrial Internet platform construction and operation, industrial intelligent technology research and application, industrial software and application development, intelligent factory construction, hardware-software integration services, and digital resource allocation for procurement, supply, and sales. It caters to industries such as home appliances, energy, healthcare, clothing, equipment, electronics, and automotive, providing services such as intelligent manufacturing and digital innovation. Additionally, it offers digital management and comprehensive service platform construction for industrial parks and regional governments, along with industrial consulting and planning services.
COSMOPlat is a productized solution refined based on Haier's interconnected factory practices and is exportable to empower small and medium-sized enterprises in their digital transformation. It aids interconnected factories in achieving “Three Connects and Three Upgrades”. Through the means of flexibility, digitization, and intelligence, the interconnected factories achieve the “Three Connects”, namely the interconnection of all elements in the “man-machine-material-method-environment” full-factor interconnection, interconnection between the network and users, and full-process interconnection. Under the ecological brand strategy, Haier’s management system is transitioning into a “Three Life System”, encompassing an ecological circle, ecological income, and ecological brand. The ecological circle is a community economy mandated by the IoT; ecological income is the experiential economy reflected by the IoT, and the ecological brand signifies the sharing economy. The ecological brand, centered on co-creation and shared user experiences, necessitates collaborative efforts from multiple partners. Taking the Tianjin Haier Washing Machine Interconnected Factory, honored with the WEF “Lighthouse Factory” accolade, as an example, COSMOPlat collaborated with ecological resources to build an ecosystem platform, integrating 5G, IoT, automation, and big data analysis technologies. This integration increased product design speed by 50%, reduced defects by 26%, and lowered unit energy consumption by 18%, achieving “high efficiency under high-precision control”. Using a self-developed IoT platform, COSMOPlat provides IoT, data collection, storage, and value-added services based on big data analysis for various intelligent terminals, creating an “AIoT end-cloud integrated” solution.
Haier has established a national-level industrial Internet platform, demonstrating its commitment not only to its intelligent construction but also to cross-industry, cross-disciplinary intelligent transformation solutions. Under the strategic direction of ecological branding, Haier is constructing a scene-driven ecosystem to lead digital transformation, utilizing its strength to build an intelligent manufacturing ecosystem.
Industry 5.0
Industry 5.0 emphasizes a people-centric approach, with a focus on employees at Haier. The shift in the philosophy from “shareholder first” to “employee first” underscores the belief that shareholders can only share profits but cannot create value. Prioritizing employees means aligning the values of employees and users, where employees can create value for users, and, in turn, shareholder value is realized. Shareholder value is seen as an outcome, not the ultimate goal.
Haier’s “RenDanHeYi” is an exploratory model with six elements that differ significantly, even disruptively, from traditional enterprises. These six elements include enterprise purpose, management model, organizational structure, driving force, financial system, and the IoT. The management model can be defined from four perspectives: theoretical foundation, supporting platform, value proposition, and value system.
The value proposition of the Haier Industry 5.0 model emphasizes value rationality as the guiding principle, advocating for individuals to become “masters of themselves”. Employees can realize their value by creating value; without creating value, they have no intrinsic value. The value proposition of traditional management models accentuates instrumental rationality, reflecting X and Y theories. X theory posits human nature as inherently bad, while Y theory posits human nature as inherently good, corresponding to the assumptions of “economic man” and “social man”. From the perspective of Industry 5.0, both these models are problematic, as they are limited by the tool rationality inherent in the antagonistic relationship between management subjects and objects.
The supporting platform of the Haier Industry 5.0 model is a parallel and versatile platform, exemplified by Haier’s large-scale customization platform. Stakeholders, such as enterprises, users, and suppliers, collaborate on the same platform, forming a co-creation and sharing ecosystem - a multi-sided platform. The era of the IoT is undoubtedly characterized by ecosystem competition, thriving only when all stakeholders benefit. Haier’s “Three Stores in One” approach, combining offline, online, and micro-stores, transforms into a community ecosystem. Haier's IoT finance embodies both community and sharing economies. The organizational structure of the platform primarily includes intelligent units, intelligent systems, and systems of intelligent systems (SoSs). Intelligent units represent the smallest units of Industry 5.0 economic growth, centered around people, information systems, and physically integrated units forming an individual-level HCPS. Intelligent systems are system-level HCPS formed by integrating multiple intelligent units through the Industrial Internet, such as intelligent production lines, workshops, factories, and enterprises. SoSs integrate across systems, platforms, and industries through the Industrial Internet, creating system-level HCPS [Figure 4], such as intelligent supply chains, industrial chains, industries, and societies.
Figure 4. New generation intelligent manufacturing based on HCPS. HCPS: Human-cyber-physical system.
The value system of the Haier Industry 5.0 model is a system and mechanism that harmoniously coordinates value creation, value transmission, and value. Each individual and user being connected transforms the traditional serial processes into parallel processes, with each parallel node creating value for users and each node realizing its value in creating value for users. This coordinated system abolishes the KPI assessments that most companies worldwide use and innovates a two-dimensional matrix of vertical and horizontal matches. The horizontal axis represents product value, with scales for high growth, high market share, and high profitability. The critical aspect is the vertical axis, with scales for the explosive power of experiential iteration, the ecosystem of community co-creation and sharing, and ecological income. First and foremost is the experiential iteration, emphasizing continuous interaction with users and iterative improvements based on user experiences. This scale does not focus on sales volume but evaluates the number of iterations. Haier introduces an innovative win-win value-added table, which emphasizes not only product revenue but also ecological income, with the goal of ecological income exceeding product revenue. Product revenue follows the law of diminishing marginal returns, while ecological income can exhibit increasing marginal returns. Haier’s digital intelligence ecological development path is presented in Figure 5.
Figure 5. Haier’s digital intelligence ecological development path. CRM: Customer Relationship Management; CAD: computer aided design; HGVS: Global Information Value-added System; ERP: enterprise resource planning; CSP: Content Security Policy; RFID: radio-frequency identification; SQL: Structured Query Language; IoT: Internet of Things; BIM: Building Information Modeling; HCPS: human-cyber-physical system.
Industry 5.0 and Society 5.0
According to the “Fifth Science and Technology Basic Plan (2016-2020)” approved by the Japanese Cabinet in January 2016, “Society 5.0” or the “Super Smart Society” represents a societal form where “necessary products and services are provided to necessary individuals at the necessary times, ensuring complete satisfaction of diverse social needs for every individual, irrespective of age, gender, location, language, etc. This realization aims to create a comfortable and vibrant society”. In the 2023 Social Responsibility and ESG Awards, the “2023 China Manufacturing Listed Companies Social Responsibility Five-Star Gold Award List” was announced, and Haier Smart Home was honored with the “2023 China Manufacturing Listed Companies Social Responsibility Five-Star Gold Award”. This recognition underscores Haier Smart Home’s steadfast commitment to the principles of Industry 5.0 and Society 5.0.
The concept of prioritizing employees holds universal applicability across diverse cultures. Haier’s approach to acquiring foreign companies involves not dispatching management personnel from the headquarters. Instead, the strategy revolves around transforming the acquired company’s principles and culture, achieving a turnaround from losses to profits. This approach is referred to as “salad bowl” cultural integration. Similar to a vegetable salad where different vegetables represent the acquired company’s original culture, retaining the original culture ensures better satisfaction of local social needs. In the salad, each component maintains its original form, while the dressing is unified, embodying the essence of “RenDanHeYi”. Haier’s Industry 5.0 culture has helped the company develop well overseas, beyond the scope of digital technology.
CONCLUSIONS
Research conclusions
The multi-case analysis results reveal the resource allocation required by enterprise digital transformation (study 1). Single-case analyses identify the procedural mechanisms and critical capabilities involved in the digitized and ecological transformation (study 2).
Multi-case configuration analysis reveals diverse paths in resource allocation
Various combinations of enterprise resources form four distinct paths, namely the growth-oriented small, the stagnant mid to large-sized, the research-deficient, and the low-profit enterprise digitization transformation paths. For different types of manufacturing enterprises, there exists a resource allocation bias concerning enterprise resources (enterprise profitability, R&D expense ratio, growth ability, company size, and human capital). The promotion of digitized and ecological transformation is facilitated through the coordinated and collaborative utilization of resources. A comparative analysis of the four paths reveals the consistent presence of human resources. Therefore, the elevation of human capital proficiency stands out as an indispensable and pivotal element in the digitized and ecological transformation.
The Haier Case: a process model for Industrial 5.0 restructuring
The Haier Lighthouse Factory provides a viable process model for the transformation of manufacturing towards Industrial 5.0, characterized by the planning path of “Standardization - Online - Integration - Intelligence - Platformization”. Standardization focuses on implementing modularity and standardization, aiming to achieve lean production. Digitalization emphasizes the establishment of network transmission and information systems to realize both production and management functions online. Simultaneously, Integration emphasizes the interconnection of all things. In addition to intelligent devices, acquiring many data points requires the intervention of sensors and radio frequency technology. Building upon standardized processes, the focus is on comprehensive data digitization throughout the entire production process. The key to IoT lies in the interconnection of all things, requiring a unified deployment for device interfaces and protocols to be effective. On the user end, efforts are made to establish platforms for user information and post-sales information acquisition, facilitating the gathering of marketing-related data. Subsequently, the introduction of big data algorithms enhances data value, utilizing big data technology to explore the inherent value of data. Based on feedback and experimental results derived from data, continuous iteration and optimization of product design and functionality are achieved, thereby attaching value to products and services and realizing the restructuring of the value chain. After the successful transformation of the enterprise with a certain level of digital capabilities, the establishment of an interconnected ecosystem enables the mutual sharing of digital resources, extending the reach of internal digital resources externally. This facilitates the promotion of service-oriented transformation and the exploration of new business formats.
The Lighthouse Factory Case reveals the mechanisms and key capabilities of Industrial 5.0 restructuring in manufacturing
(1) Digital intelligence and ecological technology drive the Industry 5.0 transformation of manufacturing with data. The digitization and ecologization technologies also assist manufacturing enterprises in achieving refined management of the production process, enhancing production efficiency and product quality. Simultaneously, with the empowerment of service capabilities by digital technologies, there is an extension of product and industrial value, restructuring the value chain and providing value-added services from technology to products and industries. The standardization, online, and integration stages realize the acquisition and circulation of full data. The introduction of intelligent equipment and the use of big data algorithms can realize intelligent analysis and scientific decision-making of data, realize intelligent decision-making and production line automation driven by data, further utilize the value of data, and transfer the value of data to the product itself and services, and further reconstruct the value chain. In other words, these technologies, driven by data, propel the transformation of the manufacturing industry, reconstructing the value chain. The objective regularity of enterprise digital transformation is guided by data value, following the path of “Standardization - Online - Integration - Intelligence - Ecologization”, progressively constructing capabilities for intelligent transformation.
(2) Digitization and ecologization technologies propel the servitization of the manufacturing industry. These technologies empower manufacturing enterprises to adopt highly flexible manufacturing, resulting in personalized customization services. Moreover, they facilitate prompt handling of user feedback. Manufacturing companies can employ IoT technology for remote monitoring and maintenance of products, enhancing product reliability and availability. By applying big data analytics, these technologies enable the analysis of customer usage patterns and preferences, providing personalized services. AI technology can be leveraged for intelligent forecasting and optimized production planning, leading to increased production efficiency and resource utilization. Digitization and ecologization technologies further assist manufacturing enterprises in achieving service customization and refinement. Using digitization and ecologization technologies, particularly through user platforms, manufacturing companies can gain in-depth insights into and analyze customer needs. This enables the provision of personalized products and services, fostering differentiation in competitive strategies. The transformation towards servitization can create new business models and revenue streams for enterprises. Through innovation driven by data, companies can integrate products with services, offering value-added services and solutions, thereby realizing diversified income streams and innovative business models.
(3) Digitization and ecologization technologies redefine the critical capabilities of the value chain, encompassing digital production capability, data-driven capability, and ecological economic capability. Digital production capability involves the incorporation of advanced technologies such as sensors, the IoT, big data analytics, and AI to establish interconnected factories and intelligent production lines, achieving highly agile production capabilities and transitioning to mass customization models. This capability serves as the cornerstone of the digitization and ecologization transformation, representing the fundamental production capability of innovative enterprises. Data-driven research and service models rely on digital production capability. Digital-driven transformation constitutes a paradigm shift in enterprise operational models. Built on the foundations of data layout and digital production capability, it incubates digital marketing. By collecting, analyzing, and leveraging vast amounts of data, digital-driven capabilities provide a more objective and accurate understanding of market demands, user behaviors, product performance, and other relevant aspects. Consequently, enterprises can make more informed decisions during the development process, delivering superior products and services. The key to building digital-driven capabilities lies in data layout, specifically in the acquisition and analytical capabilities of data. Ecological economic capability represents an advanced capability within the empowerment of digitization and ecologization technologies. Building upon the relatively mature state of digitization capabilities involves constructing a digital ecosystem to facilitate resource sharing and collaborative cooperation, enhance competitiveness, and foster innovation. This leverages new economic models in the manufacturing industry, including digital ecological economies based on experiential, community, and sharing economic models. Ecological economic capability aims to build corresponding ecological platforms with a willingness to coordinate and share, grounded in the foundations of digital capabilities such as digital factories and user platforms.
Perspectives
First, focus on the current stage of enterprise resource allocation, talent, and capital investment at each stage of the transformation process, alongside the acquisition of key capabilities. In terms of digital layout, establish interconnected factories and intelligent production lines on the production side and a user platform on the user side, guided by data, and build capabilities for data acquisition, analysis, and application. Allocate resources according to the corresponding path, guided by the process of “standardization-online integration-intelligence-ecologization”, gradually building three key capabilities: digital production, data-driven, and ecological economy.
Second, grasp the stage characteristics of green intelligent ecological-driven transformation, scientifically and moderately exert the key capabilities of each stage of green intelligent ecologization, promote resource arrangement actions to achieve green results, and realize the green transformation and upgrading of manufacturing enterprises. Therefore, manufacturing managers should accurately grasp their stage of green intelligent ecologization, activate key capabilities based on digital features, rationally allocate resource arrangement methods, and achieve intelligent production and the transformation of manufacturing services by building key capabilities of intelligent ecologization. This will drive the emergence of a new ecological economy and facilitate corporate transformation.
Third, leading manufacturing enterprises should not only achieve individual green intelligent ecologization but also play a crucial role in building a platform ecosystem, radiating the entire industry chain and supply chain for green intelligent ecologization development and collaborative greening. Therefore, leading enterprises should fully mobilize their resources and capabilities to leverage their green intelligent ecologization capabilities to transform the entire ecosystem, achieve the green leveraging of their resources, and ultimately lead the entire platform ecosystem to realize green value enhancement.
DECLARATIONS
Authors’ contributions
Conceptualization, supervision, and writing-reviewing and editing: Lai C
Writing-reviewing and editing, methodology, results, and discussion: Luo S
Availability of data and materials
The data supporting the findings of this study are not publicly available due to privacy concerns. However, the data are available from the corresponding author Luo S, upon reasonable request and with appropriate permission.
Financial support and sponsorship
This work is financially supported by the Major Science and Technology projects of Guangzhou Key R&D Plan in 2022 (No. 20220602JBGS04).
Conflicts of interest
Both authors declared that there are no conflicts of interest.
Ethical approval and consent to participate
Not applicable.
Consent for publication
Not applicable.
Copyright
© The Author(s) 2024.
REFERENCES
1. Zhuang C, Liu J, Zhang L. Connotation, architecture and enabling technology of Industrial 5.0. J Mech Eng 2022;58:75.
2. Cillo V, Gregori GL, Daniele LM, Caputo F, Bitbol-Saba N. Rethinking companies’ culture through knowledge management lens during Industry 5.0 transition. J Knowl Manag 2022;26:2485-98.
3. Alves J, Lima TM, Gaspar PD. Is Industry 5.0 a human-centred approach? A systematic review. Processes 2023;11:193.
4. Asif M, Searcy C, Castka P. ESG and Industry 5.0: the role of technologies in enhancing ESG disclosure. Technol Forecast Soc Change 2023;195:122806.
5. Haleem A, Javaid M, Singh RP, Suman R, Qadri MA. A pervasive study on Green Manufacturing towards attaining sustainability. Green Technol Sustain 2023;1:100018.
6. Maddikunta PKR, Pham Q, B P, et al. Industry 5.0: A survey on enabling technologies and potential applications. J Ind Inf Integr 2022;26:100257.
7. Xu X, Lu Y, Vogel-heuser B, Wang L. Industry 4.0 and Industry 5.0 - inception, conception and perception. J Manuf Syst 2021;61:530-5.
8. Ivanov D. The Industry 5.0 framework: viability-based integration of the resilience, sustainability, and human-centricity perspectives. Int J Prod Res 2023;61:1683-95.
9. Demir KA, Döven G, Sezen B. Industry 5.0 and Human-Robot Co-working. Procedia Computer Science 2019;158:688-95.
10. Liu Z, Wang H, Wei H, Liu M, Liu Y. Prediction, planning, and coordination of thousand-warehousing-robot networks with motion and communication uncertainties. IEEE Trans Automat Sci Eng 2021;18:1705-17.
11. Lou S, Hu Z, Zhang Y, Feng Y, Zhou M, Lv C. Human-cyber-physical system for Industry 5.0: a review from a human-centric perspective. IEEE Trans Automat Sci Eng 2024:1-18.
13. Hess T, Matt C, Benlian A, Wiesböck F. Options for formulating a digital transformation strategy. Mis Q Exec 2016;15:123-39. Available from: https://openurl.ebsco.com/EPDB%3Agcd%3A8%3A8694584/detailv2?sid=ebsco%3Aplink%3Ascholar&id=ebsco%3Agcd%3A115879199&crl=c. [Last accessed on 29 Apr 2024].
14. Warner KS, Wäger M. Building dynamic capabilities for digital transformation: an ongoing process of strategic renewal. Long Range Plann 2019;52:326-49.
15. Loebbecke C, Picot A. Reflections on societal and business model transformation arising from digitization and big data analytics: a research agenda. J Strategic Inf Syst 2015;24:149-57.
16. Gastaldi L, Appio FP, Corso M, Pistorio A. Managing the exploration-exploitation paradox in healthcare: three complementary paths to leverage on the digital transformation. Bus Process Manag J 2018;24:1200-34.
17. Svahn F, Mathiassen L, Lindgren R, Kane GC. Mastering the digital innovation challenge. MIT Sloan Manag Rev 2017;58:14-6. Available from: https://sloanreview.mit.edu/article/mastering. [Last accessed on 29 Apr 2024]
18. Sun X, Zhang Q. How can dynamic capabilities make sense in avoiding value co-creation traps? Manag Decis 2022;60:735-57.
19. Yoo Y, Boland RJ, Lyytinen K, Majchrzak A. Organizing for innovation in the digitized world. Organ Sci 2012;23:1398-408.
20. Nambisan S, Wright M, Feldman M. The digital transformation of innovation and entrepreneurship: progress, challenges and key themes. Res Policy 2019;48:103773.
21. Nambisan S. Digital entrepreneurship: toward a digital technology perspective of entrepreneurship. Entrep Theory Pract 2017;41:1029-55.
22. Nambisan S, Zahra SA, Luo Y. Global platforms and ecosystems: Implications for international business theories. J Int Bus Stud 2019;50:1464-86.
23. Ojala A, Evers N, Rialp A. Extending the international new venture phenomenon to digital platform providers: a longitudinal case study. J World Bus 2018;53:725-39.
24. Coreynen W, Matthyssens P, Van Bockhaven W. Boosting servitization through digitization: pathways and dynamic resource configurations for manufacturers. Ind Mark Manag 2017;60:42-53.
25. Vendrell-herrero F, Bustinza OF, Parry G, Georgantzis N. Servitization, digitization and supply chain interdependency. Ind Mark Manag 2017;60:69-81.
26. Kamalaldin A, Linde L, Sjödin D, Parida V. Transforming provider-customer relationships in digital servitization: a relational view on digitalization. Ind Mark Manag 2020;89:306-25.
27. Kohtamäki M, Parida V, Patel PC, Gebauer H. The relationship between digitalization and servitization: the role of servitization in capturing the financial potential of digitalization. Technol Forecast Soc Change 2020;151:119804.
28. Paiola M, Gebauer H. Internet of things technologies, digital servitization and business model innovation in BtoB manufacturing firms. Ind Mark Manag 2020;89:245-64.
29. Chen Y, Visnjic I, Parida V, Zhang Z. On the road to digital servitization - the (dis)continuous interplay between business model and digital technology. Int J Oper Prod Manag 2021;41:694-722.
31. Al-debei MM, Avison D. Developing a unified framework of the business model concept. Eur J Inf Syst 2010;19:359-76.
32. Zott C, Amit R, Massa L. The business model: recent developments and future research. J Manag 2011;37:1019-42.
33. Baden-fuller C, Haefliger S. Business models and technological innovation. Long Range Plann 2013;46:419-26.
34. Schneider P. Managerial challenges of Industry 4.0: an empirically backed research agenda for a nascent field. Rev Manag Sci 2018;12:803-48.
35. Foss NJ, Saebi T. Fifteen years of research on business model innovation: how far have we come, and where should we go? J Manag 2017;43:200-27.
36. Kohtamäki M, Parida V, Oghazi P, Gebauer H, Baines T. Digital servitization business models in ecosystems: a theory of the firm. J Bus Res 2019;104:380-92.
37. Hanafizadeh P, Mehrabioun M, Badie K, Soofi JB. A systemic framework for business model design and development -Part A: theorizing perspective. Syst Pract Action Res 2018;31:437-61.
38. Rayna T, Striukova L. From rapid prototyping to home fabrication: how 3D printing is changing business model innovation. Technol Forecast Soc Change 2016;102:214-24.
39. Sjödin D, Parida V, Palmié M, Wincent J. How AI capabilities enable business model innovation: scaling AI through co-evolutionary processes and feedback loops. J Bus Res 2021;134:574-87.
Cite This Article
Export citation file: BibTeX | RIS
OAE Style
Lai C, Luo S. Employee-centered Industry 5.0 - Case analysis of Haier. Green Manuf Open 2024;2:9. http://dx.doi.org/10.20517/gmo.2023.122601
AMA Style
Lai C, Luo S. Employee-centered Industry 5.0 - Case analysis of Haier. Green Manufacturing Open. 2024; 2(2): 9. http://dx.doi.org/10.20517/gmo.2023.122601
Chicago/Turabian Style
Lai, Chaoan, Shiban Luo. 2024. "Employee-centered Industry 5.0 - Case analysis of Haier" Green Manufacturing Open. 2, no.2: 9. http://dx.doi.org/10.20517/gmo.2023.122601
ACS Style
Lai, C.; Luo S. Employee-centered Industry 5.0 - Case analysis of Haier. Green. Manuf. Open. 2024, 2, 9. http://dx.doi.org/10.20517/gmo.2023.122601
About This Article
Copyright
Data & Comments
Data
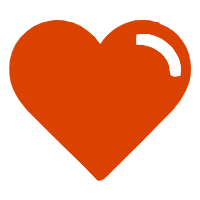

Comments
Comments must be written in English. Spam, offensive content, impersonation, and private information will not be permitted. If any comment is reported and identified as inappropriate content by OAE staff, the comment will be removed without notice. If you have any queries or need any help, please contact us at support@oaepublish.com.